Machine Learning is transforming the way we approach data and decision-making. It uses algorithms to allow computers to learn and make predictions or decisions without being expressly programmed.
In this article, we will explore what Machine learning is, its importance, and the way in which it works. We’ll break down the process of machine knowledge into clear steps, discuss various types of knowledge methods, and examine its impact on key industries like healthcare, finance, and transportation.
Toward the finish of this article, you’ll have a solid understanding of how machine learning operates, the various types of knowledge approaches, and the critical impact it has on our daily lives. This knowledge will help you value the vast possibilities of AI in different sectors.
Machine Learning
Machine learning (ML) is a branch of man-made reasoning (AI) that focuses on building systems capable of knowledge from data. In contrast to traditional programming, where unequivocal instructions are given to solve an issue, AI enables systems to perceive patterns and make decisions in light of those patterns. It adapts and works on its performance with experience over the long run.
Importance of Machine Learning
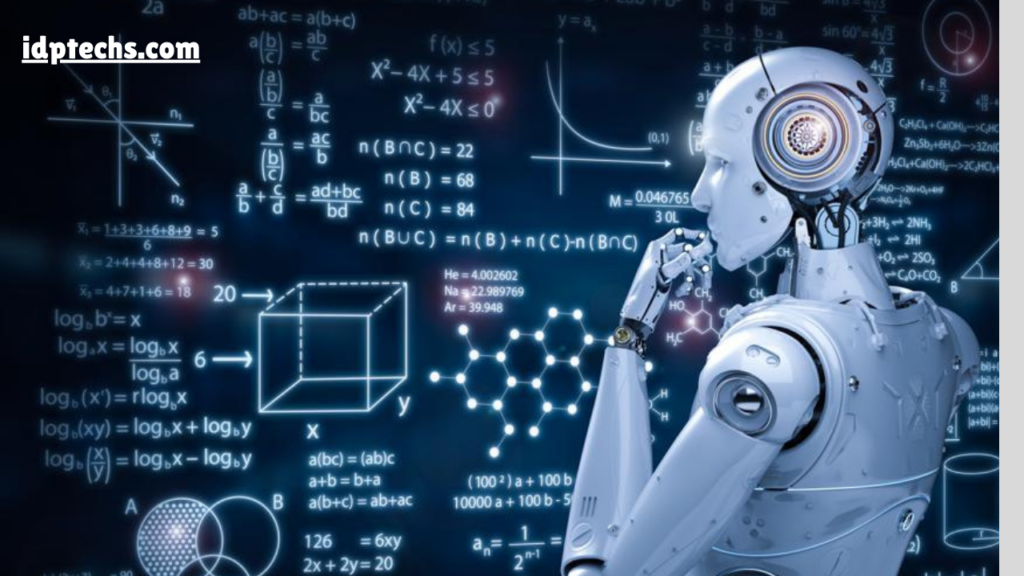
Machine learning has turned into a powerful tool for solving complex problems in different fields. Its ability to process vast amounts of information rapidly and productively helps organizations make informed decisions, figure trends, and personalize user encounters. From improving healthcare diagnoses to powering suggestion engines in online business, AI is at the core of numerous innovative solutions today.
One of the main benefits of AI is its ability to learn and improve without human intervention. As it continues to develop, it opens up additional opportunities for automation, cost savings, and improved performance across industries.
How does Machine Learning Work?
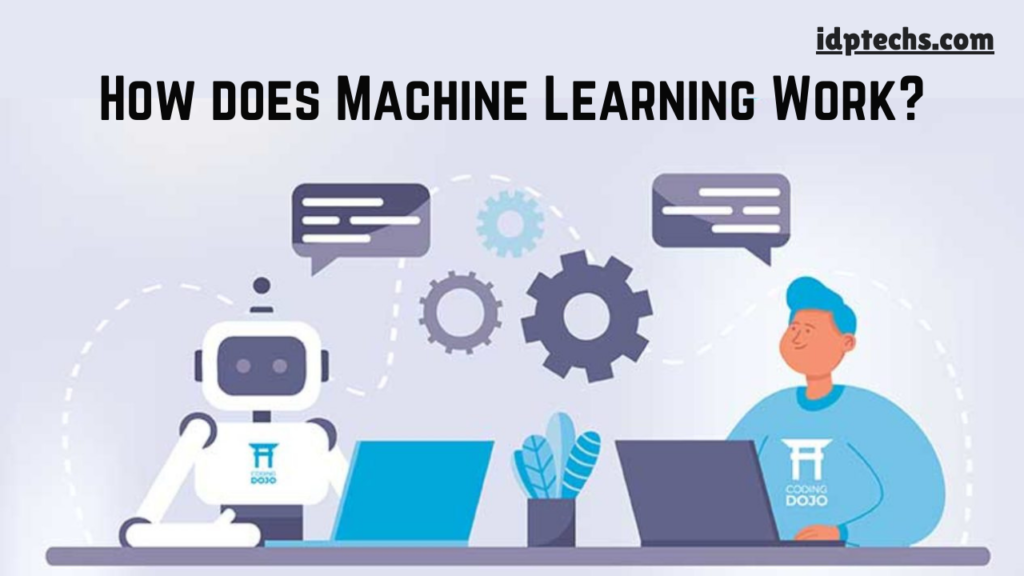
The machine knowledge process involves a few key steps. Each step plays an urgent role in ensuring that the model is accurate, reliable, and able to play out its intended task.
- Data Assortment
The most important phase in AI is gathering pertinent data. information is the underpinning of any AI model. Whether it’s text, pictures, or mathematical information, the quality and amount of the information gathered altogether impact the model’s performance.
- Data Preprocessing
In the wake of collecting the data, it should be cleaned and ready. This involves removing copies, handling missing qualities, and transforming the information into a suitable configuration for the AI calculation. Information preprocessing is fundamental for ensuring that the model can comprehend and gain from the input data.
- Choosing the Right Model
Selecting the suitable model for the task is basic. Various types of models are available, for example, decision trees, brain networks, and backing vector machines. The model ought to be picked in view of the idea of the data and the issue being solved.
- Training the Model
When the data is prepared, the following stage is training the model. During training, the model gains patterns from the information. It changes its internal boundaries to make accurate predictions or characterizations.
- Evaluating the Model
In the wake of training, assessing the model’s performance is fundamental. This is done using measurements like exactness, accuracy, and review. The objective is to guarantee that the model performs well on new, inconspicuous information and can sum up its knowledge to various circumstances.
- Hyper parameter Tuning and Enhancement
To additionally work on the model’s precision, hyper parameters (model-explicit settings) can be fine-tuned. This streamlining process involves adjusting factors like knowledge rate and regularization strategies to upgrade performance without overfitting the information.
Types of Machine Learning
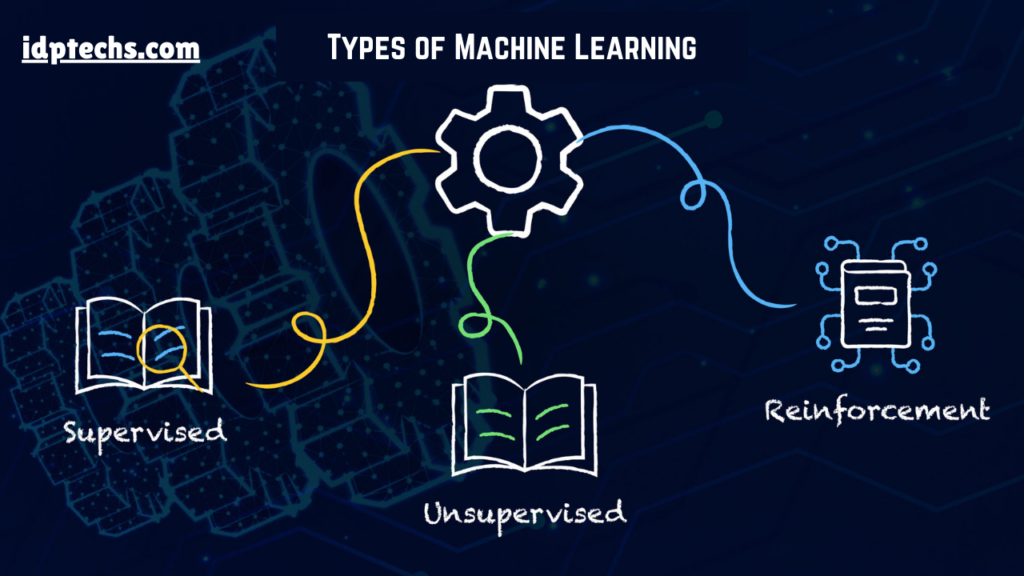
Machine knowledge can be divided into three main types, depending on how the model learns from the information.
Supervised Learning
Supervised knowledge involves training a model using labeled data. For this situation, the input information is paired with the correct output, and the model learns to map inputs to the correct outputs. It is regularly used in classification and relapse tasks, for example, email filtering or predicting house prices.
Unsupervised Learning
In unsupervised knowledge, the model works with data that has no labels. It recognizes patterns and connections within the information without predefined replies. Clustering and affiliation are normal tasks in unsupervised knowledge. It’s helpful in market segmentation or client conduct analysis.
Reinforcement Learning
Reinforcement knowledge involves training a model through interactions with its environment. The model learns by trial and error, receiving compensations for effective actions and penalties for mistakes. This sort of knowledge is much of the time used in gaming, robotics, and autonomous systems.
Impact of Machine Learning
Machine knowledge is having a transformative impact on a large number of industries. We should explore three sectors where its impact is especially noticeable.
Healthcare
In healthcare, AI is revolutionizing diagnostics and treatment. For instance, ML algorithms can analyze radiology scans to detect tumors or predict patient results based on historical information. This helps doctors make quicker and more accurate decisions, improving patient results.
Finance
The finance industry depends vigorously on AI for fraud detection, algorithmic trading, and risk the board. Also, AI helps financial institutions predict stock prices, mechanize trading procedures, and assess credit risk all the more actually.
Transportation
Machine knowledge is additionally making waves in the transportation industry. It’s used to enhance traffic flow, predict vehicle maintenance needs, and work on autonomous driving systems.
For instance, ride-hailing services use AI to predict demand and adjust prices powerfully. Autonomous vehicles use reinforcement knowledge to explore complex environments, making transportation more secure and more efficient.
Conclusion
In this article, we explored the idea of machine learning, its importance, and the key steps involved in creating an AI model. We discussed the various types of AI, including supervised, unsupervised, and reinforcement knowledge, as well as their applications.
AI is a powerful tool that continues to shape the future of technology and innovation. As it develops, its ability to solve complex problems and further develop decision-making will just increase. Understanding its basics and the way in which it tends to be applied in different sectors gives valuable insight into the future of AI-driven solutions.
FAQ’s
1. What is the distinction between machine learning and AI?
AI is a subset of AI focused on systems knowledge from data. AI covers a more extensive scope of innovations that mimic human intelligence. Machine knowledge explicitly manages improving performance through experience.
2. What are a few normal utilizations of machine learning?
AI is used in proposal systems (e.g., Amazon), fraud detection in banking, and healthcare diagnostics. It’s additionally applied in autonomous vehicles and menial helpers like Siri and Alexa.
3. How much data do I really want for machine learning?
The amount of data required relies upon the issue’s intricacy. More information further develops accuracy, however methods like exchange knowledge can work with more modest datasets. Complex models might require a huge number of information points.
Hello. . nw.dn.ua
nw.dn.ua
https://nw.dn.ua zwz4763574